
Date: Mon Jun 25, 2018
Time: 1:30 PM - 3:00 PM
Moderator: Yuxin Miao
Improving corn (Zea mays L.) nitrogen (N) fertilizer rate recommendation tools should increase farmer’s profits and help mitigate N pollution. Weather and soil properties have repeatedly been shown to influence crop N need. The objective of this research was to improve publicly-available N recommendation tools by adjusting them with additional soil and weather information. Four N recommendation tools were evaluated across 49 N response trials conducted in eight U.S. states over three growing seasons. Tools were evaluated for split (planting+side-dress) fertilizer applications. Using an elastic net algorithm the difference between each tool’s N recommendation and the economically optimum N rate (EONR) was regressed against soil and weather information, then the elastic net regression coefficients were used to adjust the tool’s N recommendation. The evenness of rainfall calculated from planting to the date of sidedness and soil pH (0-0.30 m) were the most frequently identified parameters for adjusting tools. All tools showed improvement with adjustment (+r2 ≥ 0.09). The greatest improvement in tool performance was with including soil and weather information with the Late-Spring Soil Nitrate Test (LSNT), canopy reflectance sensing, and MRTN. This analysis demonstrated that incorporating soil and weather information can help improve N recommendations.
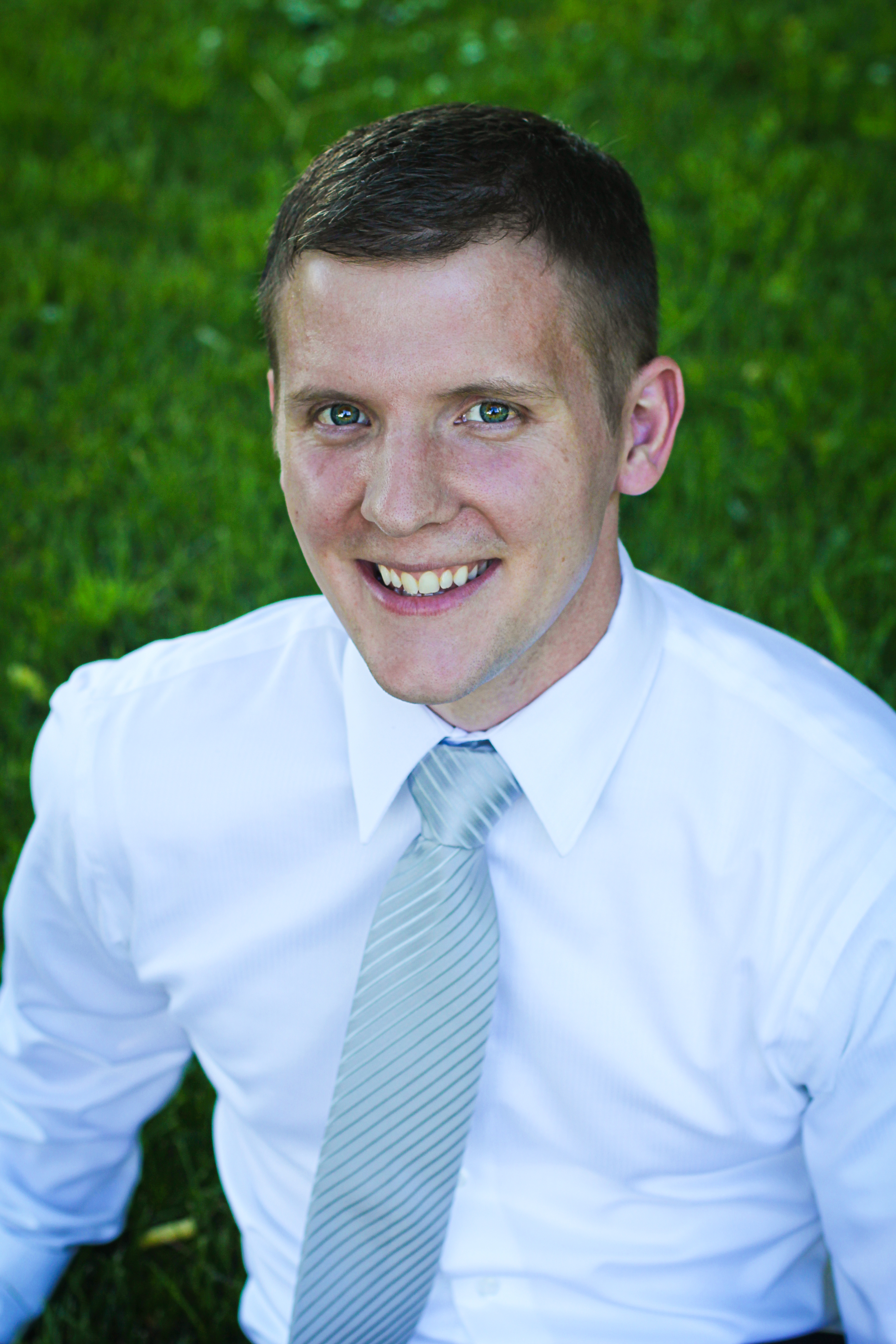
The relative cost of Nitrogen (N) fertilisers in a cropping input budget, the 33% Nitrogen use efficiency (NUE) seen in global cereal grain production and the potential environmental costs of over-application are leading to changes in the application rates and timing of N fertiliser. Precision agriculture (PA) provides tools for producers to achieve greater synchrony between N supply and crop N demand. To help achieve these goals this research has explored the use of management classes derived from historic field data and in-season crop reflectance sensors in an attempt to quantify, and manage the effects of, spatial and temporal variation in N uptake. This simple study combines the two techniques to try and quantify in-season variation in N requirements, and furthermore attempts to improve the predictive ability of in-season yield prediction functions through the inclusion of historic soil and yield data sets. Experiments from two example fields are used to quantify seasonal variations in N using in-season reflectance data. A process was designed to build site-specific N requirement algorithms from reflectance and historic input data. The variation in historic yields and current season reflectance indices across potential management classes indicates that the magnitude of variation in plant N requirements is sufficient to implement management classes in conjunction with in-season crop reflectance sensors. Furthermore the development of modified site-specific yield prediction functions according to management classes built from soil ECa data, previous yield observations and calibrated yield prediction functions significantly enhanced yield prediction accuracy. These improved in-season yield predictions were used to construct N application strategies that proved more cost effective than traditional approaches. The combination of site-specific historic data and in-season reflectance information shows promise for the development of N application decision support to improve NUE in both economic and environmental terms.
Optimizing nitrogen (N) fertilization is important to improve corn yield and to reduce N losses to the environment. The economic optimum nitrogen rate (EONR) is variable and depends on many factors, including weather conditions and crop management. The main objective of this study was to examine how grain corn yield response to N varies with planting date, soil texture and spring weather across sites and years in Monteregie, which is the most important with 64% of total area and 69% of total harvested grain for this crop in Quebec province. This experiment was conducted during 8 years (2002 to 2004 and 2006 to 2010), at 11 sites with 23 hybrids and four N applications rates, for a total of 45 site-years. Each six year involved five and six rates ranging from 80-90 to 240 kg N ha-1. Trials were separated into two groups based on optimal and late planting dates. Grain yield response to N varied among site-years. Significant differences in grain yield among the applied N rates were observed in all of the site-years planted at optimal dates (from 8.8 to 14.7 Mg ha-1), and in most of those planted late (8.5 to 12.8 Mg ha-1). Overall, optimal planting window increased grain yield compared to late planting. EONR ranged less widely for site-years planted on optimal dates (180 to 237 kg N ha-1) than for those planted late (132 to 237 kg N ha-1). Soil textural classes and rainfall affected the EONR. The average EONR was 193 and 204 kg N ha-1for fine-textured soil and coarse-textured soil, respectively. Response to fertilizer N was higher for early planted corn combined with wet conditions. These results suggest that the current N guideline may need to increase for the optimal planting window and should be based on soil texture and weather.
Corn nitrogen recommendations for individual fields must improve to minimize the negative influence that agriculture has on the environment and society. Two adaptive N management approaches for making in-season N fertilizer recommendations are remote sensing and crop systems modeling. Remote sensing has the advantage of characterizing the spatial variability at a high spatial resolution, and crop models are prognostic and can assess expected additions and losses that are not yet reflected by the plant (e.g., due to recent management, weather, etc.). Remote sensing can be used to estimate crop biophysical parameters such as leaf area index or biomass, and can be used to calibrate crop systems models for making more accurate N fertilizer recommendations. A challenge in implementing this, however, is that an independent model calibration is required for each spatial area to be modeled. This study aims to test an autocalibration method at the sub-field scale for use in calibration of the EPIC (Environmental Policy Integrated Climate) model so it can be used more reliably for precision agriculture applications. EPIC is capable of simulating crop growth, nutrient transport and demand, and water movement on a daily time step. Following an initial calibration, an independent calibration is performed for each area within the study area using spatially precise outputs derived from remote sensing data (i.e., leaf area index and biomass). A field experiment was conducted in 2017 with four nitrogen rates and two timings of N fertilizer (i.e., preplant and V5 leaf stage). Tissue N and aerial imagery were collected at several days during the early growth stages to use as a basis for implementing and testing the autocalibration approach. The Monte Carlo algorithm was used to generate samples for the autocalibration step, and the Nash-Sutcliff efficiency was used as an objective function to analyze and interpret the results. The techniques utilized in this study serve as a framework for being able to use crop systems models for making more accurate N fertilizer recommendations.
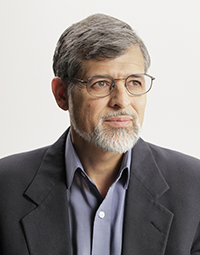
Conventional nitrogen (N) management for potato production in the Upper Midwest, USA relies on using split-applications of N fertilizer or a controlled release N product. Using remote sensing to adaptively manage N applications has the potential to improve N use efficiency and reduce losses of nitrate to groundwater, which are important regional concerns. A two-year plot-scale experiment was established to evaluate adaptive N-management using remote sensing compared to conventional practices for Russet Burbank variety potatoes grown on an irrigated, coarse-textured soil in Becker, MN. Nitrogen treatments included (Control) a 45 kg N/ha control treatment, (270 Split) a splitapplied urea treatments of 270 kg N/ha, (270 CR) a controlledrelease polymer coated urea [PCU] treatments of 270 kg N/ha, and (VR Split) a variablerate splitapplied urea treatment based on remote sensing observations using the MERIS Terrestrial Chlorophyll Index [MTCI] interpreted using the Nitrogen Sufficiency Index [NSI]. Using the CROPSCAN MSR-16R ground-based multispectral radiometer, spectral reflectance measurements were collected weekly during the growing season to monitor crop N status in the variable rate treatment (VR Split) and calculate NSI with 270 CR as the “well-fertilized” reference. If the NSI value immediately prior to scheduled fertilizer application was less than 0.95, then N-fertilizer was applied to VR Split at a rate of 22 kg N/ha. The variable-rate treatment (VR Split) received 248 and 226 kg N/ha in 2016 and 2017 respectively, which is 22 and 44 kg N/ha less than the conventional N management practices (270 Split, 270 CR), and there were no significant differences in the quantity or quality of tuber yield between these treatments. This study demonstrates that adaptive N management using remote sensing is a promising method to optimize N rate and timing to account for spatial and temporal variability in crop N status for potato production.
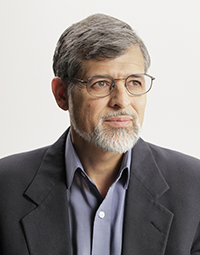
This paper describes a practical approach for the prescription of variable rate fertilization maps using remote sensing data (RS) based on satellite platforms, Landsat 8 and Sentinel-2 constellation. The methodology has been developed and evaluated in Albacete, Spain, in the framework of the project FATIMA (http://fatima-h2020.eu/). The global approach considers the prescription of N management prior to the growing season, based on a spatially distributed N balance. Although the diagnosis of N status showed promising results, the necessity of prognostic tools for N management is undiscussed for crops like wheat. The spatially distributed N balance relies in the use of management zone maps (MZM) based on temporal series of RS data. The MZMs are calculated for previous growing seasons, based on the up-to-date RS approaches to estimate yield and biomass, and captures the within-field variability of crop production. Thus, the classical N balance model is used to calculate the N requirements at pixel scale, varying the soil properties when the input data are available and the target yield according to the MZM. It has been implemented trials and demonstration activities in commercial fields in the study area, analyzing the performance of the proposed fertilization strategies. The preliminary results indicated the possible optimization of the N application, maintaining or increasing the crop productivity and reaching the higher levels of yield quality in the area.
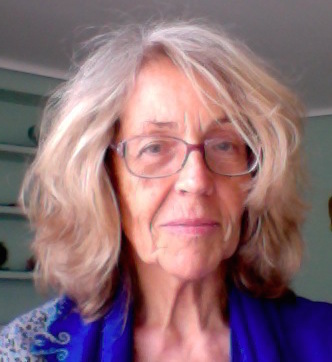